Unveiling Bias in Job Descriptions and Navigating Fairness in Recommender Systems
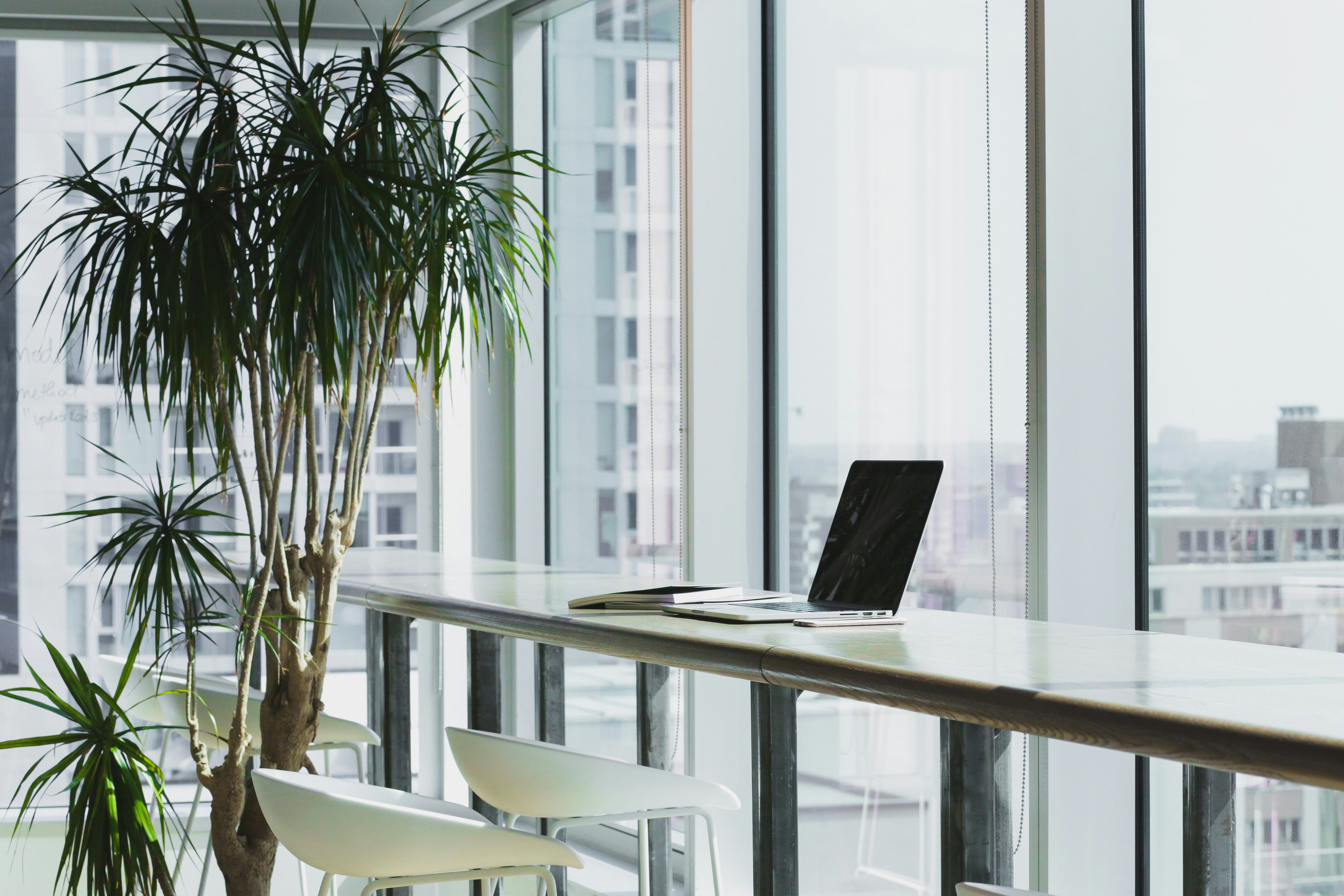
Unveiling Bias in Job Descriptions and Navigating Fairness in Recommender Systems
Recent innovations in algorithmic large language models such as the launch of Chat-GPT and Gemini to name a few, have helped to widen general awareness, by making these concepts more accessible to the general public, about bias in both data and output of Generative Artificial Intelligence. Although these models are still under development, they have the potential to revolutionise the mechanics of our interactions with technology. They can be used to create more natural and engaging user experiences, automate tasks that are currently done by humans, and generate new insights from data.
By generating human-like texts in any language, generative AI also has a large impact on Recruiting and Talent Acquisition. As in other professional domains, it can improve efficiency and streamline processes. However, especially within Human Resources, we must be aware of the risks of using artificial intelligence, ensuring that diversity, equity and inclusion are honoured and maintained instead of counteracting well-established practices in organisations.
On job boards, we must be aware of the bias in recommender systems as well as of bias in large language models in case they are used for enriching or creating content.
Where does bias come from?
All humans are biassed. Unconscious (or implicit) bias affects everyone and results from human brains being trained for thousands of years to make quick assumptions, assessments, judgments and decisions. It has a significant impact on how we react towards other human beings, which can be good, bad or neutral for an individual. So, why do we consider it a problem when Chatbots or AI are biased?
Cognitive bias is multifaceted however, it can be counteracted, e.g. by awareness training, collecting more data or making decisions in diverse groups to name a few strategies, and yet we still have a long way to go and to improve as a society. With machines and generative AI it’s not trivial to implement the same measures and many machine learning models lack explainability, meaning it’s impossible to explain why a certain input of data yielded to a certain output. Especially with AI we have seen that the quality of the data used for training the model has a high impact on the output. Data can be skewed in many directions, and a bias in training data may lead to algorithmic bias. Eventually, even cognitive bias from humans may end up in the algorithms: who trains an AI model and how it is trained has an impact, there are strong indications that more diverse teams lead to less discriminatory products.
How can we counteract discrimination on job boards?
Firstly, by establishing guidelines and rules on how to phrase content and job descriptions - no matter if they were written by humans or Artificial Intelligence. However, texts and content are not the only place where bias comes into play - it may also be in the recommender systems and/or the search engine, which should be analyzed critically as well as built and configured carefully. Among the technical solutions to reduce bias, especially in recommender systems you will find suggestions for processing data (e.g. changing the training data), fairness through unawareness (weighting solely based on the user’s actions) and post-processing (e.g. influencing a ranking to discriminate a dominant element).
In collaboration with Markus Schedl at the Institute for Computational Perception at Johanes-Kepler-University in Linz, Austria, Jobiqo is researching on possibilities to mitigate Gender Bias in Job Recommender Systems. By identifying discriminatory wordings in job descriptions (and other texts), fairness in machine learning tasks is improved and implemented in consent-debiased and fair recommender systems.
We will continue to dive deeper into this, but also into bias within large language models and introduce AI features with the necessary care to not only fulfill legal requirements but also to build a more equal and fairer future.
Learn how you can grow your Job Board with Jobiqo's white label job board software
Contact Us Now
Jobiqo enables media brands and publishers worldwide to build next-generation job boards and career marketplaces to engage talent. By combining the benefits of a scalable SaaS platform and the power of our AI-enabled Smart Matching technology, Jobiqo customers can quickly react to changing market demands and stay competitive in an ever-challenging market.
Footnotes and References
Imperial College: EDI - What is unconscious bias?
Mitigating Gender Bias in Job Recommender Systems: A Machine Learning Law-Synergy
Writing Credits: Barbara Steinkellner, Chief Operations Officer at Jobiqo
Title Photo: Unsplash; Photo by Alesia Kaz